Powering Generative AI: The Data Center Energy Challenge and Sustainable Solutions
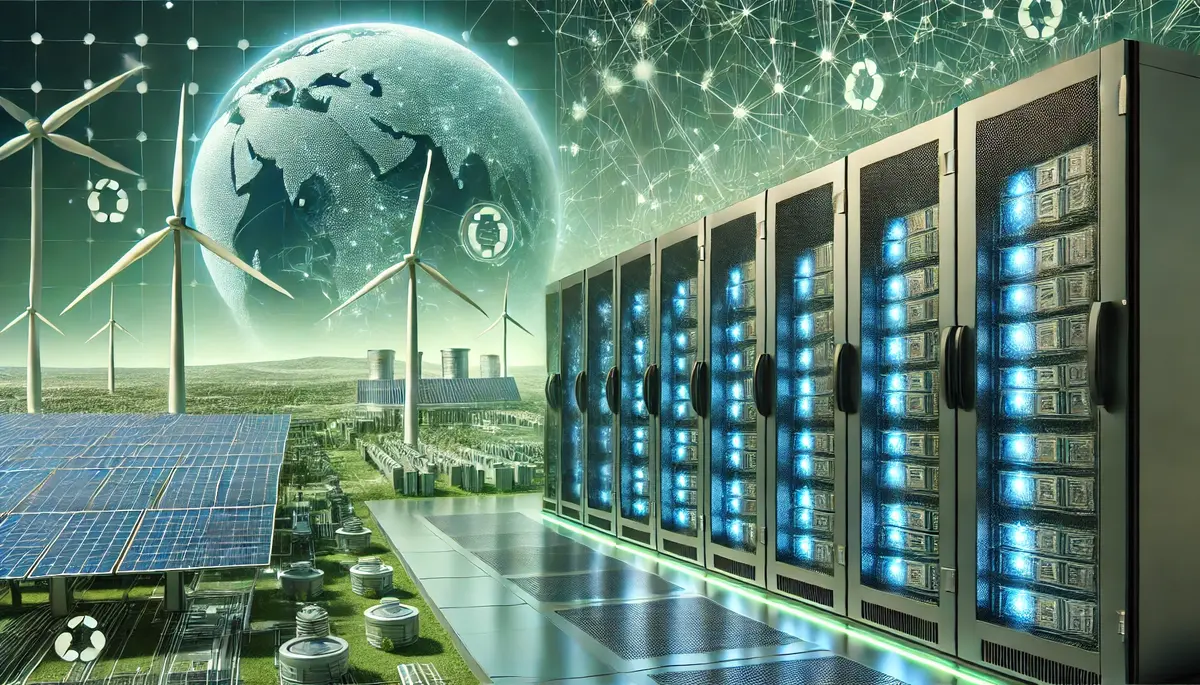
In the rapidly evolving digital landscape, generative Artificial Intelligence (GenAI) models like ChatGPT have become integral to various applications, from drafting emails to creating art. However, this technological advancement comes with a significant energy cost. A single ChatGPT query consumes approximately 0.0029 kilowatt-hours (kWh) of energy, nearly ten times the energy required for a typical Google search, which uses about 0.0003 kWh per query.
As these AI models become more prevalent, their reliance on expansive data centers intensifies, leading to increased energy consumption and environmental impact. This article explores the energy demands of GenAI, the associated environmental consequences, and strategies to promote sustainability in data center operations.
The Energy Appetite of Generative AI in Data Centers
Data centers are the backbone of AI technologies, providing the necessary infrastructure for training and deploying models. Globally, data centers consumed about 340 terawatt-hours (TWh) of electricity in 2023, accounting for approximately 1.3% of worldwide electricity use.
The energy requirements for training large language models are substantial. For instance, training GPT-3 consumed an estimated 1,287 megawatt-hours (MWh) of electricity, equivalent to the annual energy usage of over 120 homes. Moreover, the inference process—running these models to generate outputs—demands continuous computational power, further escalating energy consumption.
Read more: Driving the Future: Integrating Renewable Energy into Data Centers
Environmental Impact
The escalating energy consumption of data centers has several environmental implications:
Carbon Emissions
The substantial energy use of AI workloads contributes significantly to greenhouse gas emissions. For example, training GPT-3 resulted in the emission of approximately 502 tons of CO₂, comparable to the emissions from driving a car to the moon and back.
Water Usage
Cooling data centers necessitates considerable water resources. Training GPT-3 alone consumed an estimated 700,000 liters of water, highlighting the strain on local water supplies.
Grid Strain
The rapid expansion of data centers, especially those supporting AI applications, is outpacing the growth of grid capacity. This imbalance can lead to increased reliance on fossil fuels during peak demand periods, undermining renewable energy efforts.
Read more: Global Data Centers in 2025: The Evolution of Digital Infrastructure
Strategies to Minimize the Impact
Addressing the environmental challenges posed by AI-driven data centers requires a multifaceted approach:
1. Energy-Efficient Hardware
Transitioning to specialized hardware can significantly reduce energy consumption. Graphics Processing Units (GPUs) and Tensor Processing Units (TPUs) are designed for high-efficiency AI computations, potentially cutting energy use by up to 50% compared to traditional Central Processing Units (CPUs). For instance, Nvidia’s advanced chips aim to deliver superior performance with reduced power requirements.
2. Renewable Energy Integration
Powering data centers with renewable energy sources is crucial for reducing their carbon footprint. EDGE DC has been using 100% renewable energy to power their facilities. Companies like Google and Microsoft are also investing in geothermal and nuclear energy projects to meet the growing energy demands of their AI operations sustainably.
3. Smarter AI Design
Optimizing AI models to be more energy-efficient can substantially decrease their environmental impact. This includes reducing the number of parameters, employing smaller, task-specific models, and minimizing the frequency of retraining. Such practices not only conserve energy but also maintain or even enhance performance.
4. Advanced Cooling Techniques
Innovations in cooling technologies can lead to significant energy and water savings. Liquid-to-chip cooling and air-based systems are emerging as alternatives to traditional water-intensive methods, potentially reducing energy costs by 20-50%.
Read more: Key Features of a Hyperscale Data Center
Conclusion
The integration of GenAI into various sectors offers transformative possibilities but also presents significant energy and environmental challenges. By adopting energy-efficient hardware, integrating renewable energy sources, designing smarter AI models, implementing advanced cooling techniques, and enhancing grid collaboration, the tech industry can mitigate the environmental impact of AI-driven data centers.
As consumers and stakeholders, supporting sustainable tech practices and advocating for energy-efficient solutions are essential steps toward a more environmentally responsible digital future.
Looking for a data center facility to house your AI business? Learn more about how EDGE DC can power your AI infrastructure responsibly.